AGMRI evaluates field conditions using imagery to create valuable aerial and NDVI maps
Aerial imagery is a great first layer to view field conditions because it displays an image familiar to the eye. Utilizing the aerial layer in AGMRI, look for obvious insights like tire tracks, wet spots, residues, etc. In this case, there was an obvious streaking and lighter color of plants which often indicates a nutrient deficiency. For these types of situations, the measuring ruler is handy to help determine the width of the streaks or width between the pattern, which can give insight into application widths. AGMRI’s high- resolution imagery also enables users to zoom in and can often narrow it down to the exact row impacted.
The Issue
Using AGMRI aerial image, a farmer noticed a streaking pattern in a field. The same pattern was present later in the season on the NDVI map. The farmer used the measurement tool within AGMRI to understand the distance between the visible streaks and determined it was the same width as his side dress bar or nutrient applicator bar. Zooming further into the image, it was noted that four rows were affected. The farmer identified a clogged nozzle at the end of the toolbar.
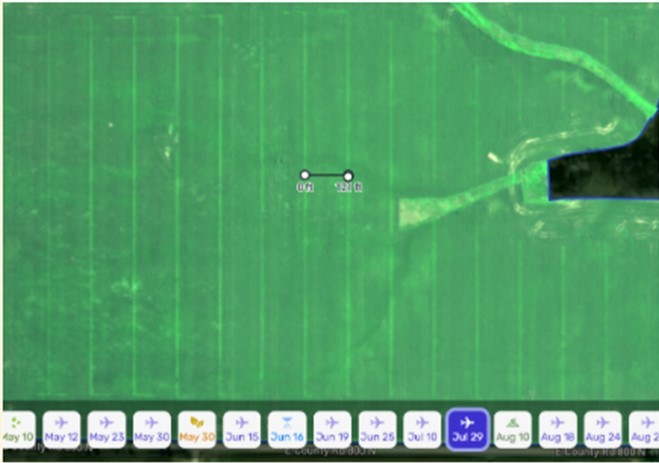
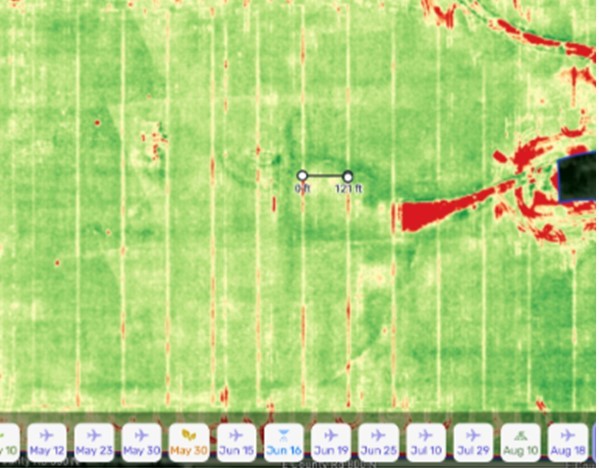
Using AGMRI to Monitor Fields
Through AGMRI, it was determined there was a clogged nozzle on the applicator used to side dress the nitrogen. The farmer used the AGMRI finding to help train his employees to conduct a thorough check of the nozzles when entering and leaving a field to prevent it from occurring in the future.
Contact us for more information or to schedule an AGMRI demo.